A re-visit to the famous Jeff Leek commentary
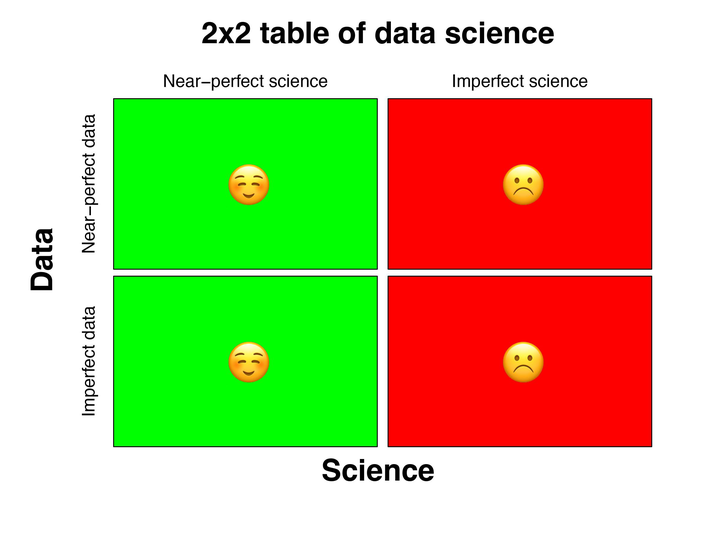
In 2013, I came across this famous blog post by Jeff Leek. Little did I know that I would revisit this post 9 years down the lane. As it turns out, it’s not a bad idea to revisit old work with fresh eyes and new perspectives, which is what I have attempted to do here based on personal experience.
Without further ado, let’s talk about a typical data science project and let’s break it down into a few possible scenarios and their consequences (hint: the reality is always in between).
Let’s first look at the diagonal elements of the oversimplified 2x2 table above:
Perfect data + perfect science: Win-win
Imperfect data + imperfect science: Lose-lose
It’s a no brainer that the combination of perfect data and perfect science is the most desirable scenario for most data scientists and decision-makers. You don’t need a sophisticated method or model when your data is near-perfect (at least most of the time) - a simple visualization or a simple model might reveal what you are looking for (or a lack thereof).
In a similar vein, the least desirable scenario is the combination of near-garbage data and near-garbage science - not much to say here except that it’s unfortunate when that happens and a lose-lose scenario for everyone involved.
Note that the above ‘garbage in garbage out’ concept is possibly one of the most discussed topics in the data science community. Yet, this concept is often overused with misleading practical implications.
Quite naturally, when a dataset is 100% garbage, the ‘garbage in garbage out’ concept clearly stands out. Period. No further discussion is necessary. In reality, however, a dataset is never 100% garbage - it can be imperfect and noisy but not 100% garbage, which is when this concept gets tricky and ends up being misconstrued.
To understand this, let’s look at the other two scenarios (off-diagonal elements in the 2x2 table above) which is typically harder than the previous ones where the ‘science’ or the ‘statistical’ aspect of ‘data science’ can no longer be taken out of the equation unlike the ‘diagonal’ use cases discussed before:
Perfect data + Imperfect science: Highly undesirable. The damage might be less visible given the rich amount of information but it’s hard to justify the missed opportunity.
Imperfect data + perfect science: Real test of a data scientist as uncertainty quantification is no longer optional. When successful, it’s a near-win for everyone involved.
As a data scientist, I thrive for a scenario when the data is imperfect which lets me test my mettle and let me break out of my comfort zone albeit at the expense of complexity, which can act as a barrier to wide acceptance and recognition. On the other hand, I have remained equally enthusiastic when the associated data is near-perfect which provided me an extra layer of cushion to posit a simpler but more impactful data science solution.
This brings me to the central point of this post. Amidst the natural human tendency to be safe, certain, and stress-free, it’s possible to forget the key word in data science which is not ‘data’ but ‘science’ even in the situation when the data is imperfect.
In many cases, this ‘science’ entails ‘advanced statistics and machine learning’ (and sometimes mathematical and computational innovations) and doing science on imperfectly collected data is astronomically harder than any of the above-mentioned ‘perfect data’ scenarios. This is when it becomes difficult to resist the temptation to oversimplify, which is exacerbated by the fact that stakeholders tend to be distrustful of the results (regardless of whether this is warranted) given the complexity of the methods involved.
To conclude, while it is necessary to err on the side of caution in light of the potential pitfalls of a premature conclusion, an exploratory and open mind outside of the ‘comfort zone’ might be necessary to answer a particularly hard question especially when the ‘data’ is imperfect which brings me to re-iterate the main point of the 2013 Jeff Leek blog post:
‘The key word in “Data Science” is not Data, it is Science’.
It is the science that can either break or make your data not the other way around :)